πGermany
Staff Data Scientist
closed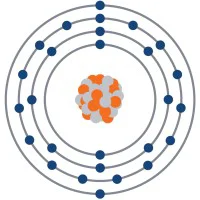
KoBold Metals
π΅ $190k-$235k
πRemote - Worldwide
Summary
Join KoBold, a leading mineral exploration company, as a Staff Data Scientist. You will build predictive models using scientific computing, statistical, and physics-based methods to locate ore deposits. This role involves developing a worldwide dataset, creating 2D and 3D geologic predictions, and collaborating with geoscientists and stakeholders. You will contribute to KoBold's proprietary software exploration tools and help guide exploration decisions. As an early team member, you'll build these tools from the ground up, accelerating the discovery of critical battery metals. This is a full-time, exempt position with a remote-first work environment.
Requirements
- Possess extensive experience with Pythonβs data science packages and general software engineering practices
- Be proficient in collaborative software development (git), and familiar with software engineering best practices like unit test / integration test suites, and CICD pipelines
- Have experience with cloud computing resources
- Have experience building predictive models, applying them to different problems, and evaluating and interpreting the results
- Have experience working with data from a variety of physical systems
- Have experience with geospatial analyses and visualizations
- Possess broad skills in and knowledge of applied statistics and Bayesian inference
- Have a substantial understanding of machine learning algorithms
- Hold an advanced degree in the physical sciences, engineering, computer science, or mathematics
- Have a minimum of 6 years post-degree work experience as a data scientist or data engineer
- Have experience leading technical teams to apply novel scientific approaches to core business problems
- Be able to design, scope and implement technical solutions to novel exploration problems
- Demonstrate the ability to quickly absorb and synthesize complex information, with a track record of high intellectual rigor in a professional setting
- Possess exceptional curiosity and eagerness to learn, with a proactive approach to exploring new concepts and technologies
- Show excitement about joining a fast-growing early-stage company, comfort with a dynamic work environment, and eagerness to take on a range of responsibilities
- Be able to independently prioritize multiple tasks effectively
Responsibilities
- Help develop KoBoldβs proprietary software exploration tools
- Find and curate geophysical, geochemical, geologic, and geographic data and integrate it into KoBoldβs proprietary data system
- Build models to make statistically valid predictions about the locations of compositional anomalies within the Earthβs crust
- Create effective visualizations for evaluating model performance and enabling rapid interaction with the underlying data and key features
- Develop and apply data processing, statistical, and physics-based techniques to geoscientific data β from computer vision to geophysical inversions β and use the results to guide our targeting efforts and inform our acquisition and exploration decisions
- Present to and collaborate with our external partners and stakeholders
Preferred Qualifications
- Have experience creating machine learning models on geospatial data
- Have experience with geostatistics
- Have experience with image processing or computer vision
- Have experience with distributed computing applications for machine learning and other computations
Benefits
- Remote-first workplace
- US base salary range for this full-time exempt position is $190,000-$235,000
This job is filled or no longer available
Similar Remote Jobs
πAustralia
π°$217k-$303k
πUnited States
π°$193k-$253k
πUnited States
π°$188k-$225k
πUnited States
π°$194k-$240k
πWorldwide
πUnited Kingdom
π°$194k-$240k
πWorldwide
πIndia
π°$190k-$235k
πWorldwide